Beyond Translation: Building Culturally Intelligent AI for India
Culture influences how we think, act, and understand the world. From the subtle nuances of a joke to shaping our core beliefs, culture acts as a lens between our experiences and perceptions. And this lens is particularly important when we consider technologies that interact with and interpret human behaviour.
As AI permeates our daily lives with interactions fluctuating from objective to subjective, understanding its cultural blind spots becomes integral.
To understand this better, let’s take the example of dal chawal—a simple, staple dish that, for many Indians, represents comfort in a bowl. Now, imagine one day someone replaced your beloved dal chawal with "curry rice."
And while you appreciate the sentiment behind the curry rice, you wish you could just replace it with nani ki dal chawal. It’s the same experience we often have when interacting with LLMs. It might not come across in the first few superficial questions, but as we begin to probe further, there is a distinct divergence and the curry rice reveals itself.
Cultural Disconnect in AI Systems
A recent study analyzing cultural values across 107 countries revealed a concerning pattern: popular AI models like GPT show strong alignment with Western cultural values, especially those common in English-speaking and Protestant European nations.
What makes this particularly problematic is how these AI models consistently favor individual expression and secular values—perspectives that often conflict with India's cultural framework shaped by inherently collectivist notions.
And this bias becomes increasingly prevalent when we start using LLMs for more subjective tasks like generating creative content to providing emotional support.
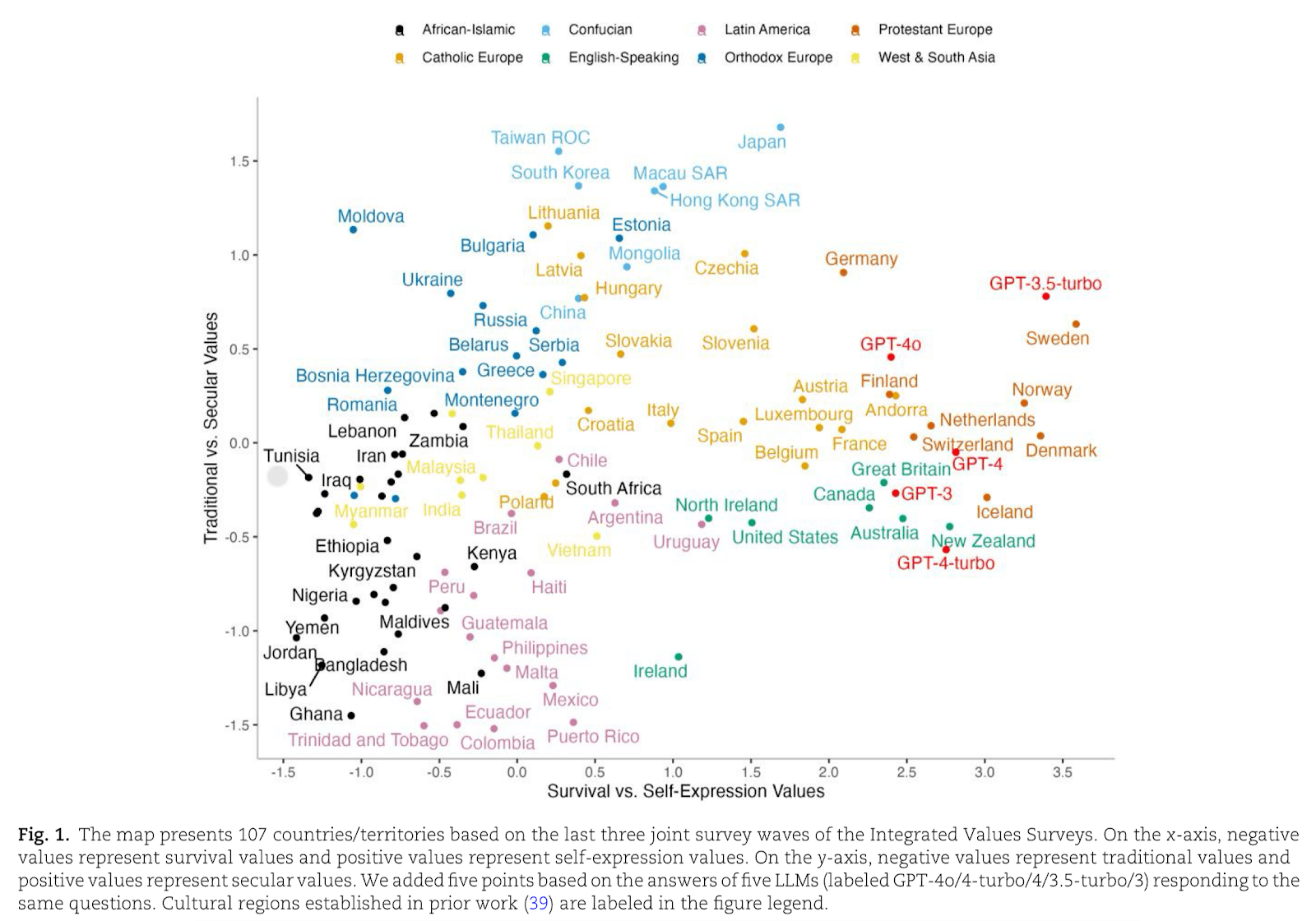
Translating language is one thing. But compressing and translating years of rich history experienced so differently in the geographic expanse of our country is a whole other. When your aunt was saying “unhe ghabrahat ho rahi hai” she was probably trying to find the words for her anxiety.
In this phrase, rests an entire culture’s need for LLMs which understand this context. We can speak about best practices, mindfulness and self care much easier as these are concepts borrowed from the West but how can we convey emotions felt in concepts and frameworks created by them?
Think about an adolescent Indian girl from Kerala and her American counterpart living in some part of Tennessee, both with working parents, contemplating whether to tell their mothers about a poor test score.
Despite similar surface circumstances, their decision trees diverge dramatically—each navigating different value systems, facing different consequences, and carrying different cultural burdens. Thus, a culturally contextual LLM needs to consider traditional beliefs, social norms, and family dynamics, among other aspects, to respond effectively.
Current Limitations in LLMs
Imagine an algorithm trained to understand human experience, but with a lens so narrow it can only see through a single, borrowed window. This limitation becomes painfully clear when we examine the cultural constraints of SOTA LLMs:
Predominantly rely on formal knowledge sources like Wikipedia and internet articles, which often miss the rich, evolving cultural nuances
Tend to present cultural knowledge in an assertive manner, failing to capture the inherent diversity within subgroups
Are trained on data that reflects human biases, which means they can accidentally propagate stereotypes
Depend on traditional evaluation methods focused on classification tasks, which do not adequately measure real-world cultural awareness
Building Cultural Understanding: A Three Layer Approach
Foundational (easily taught): This layer includes straightforward, rule-based aspects that are explicitly programmable into LLMs, such as language syntax, standard translations, and basic cultural formalities specific to India. For example, LLMs can be taught the grammatical structure of Hindi sentences, the use of respectful address such as "ji" after names to denote respect
Adaptive (learned with experience): This layer involves cultural knowledge acquired over time through social interactions within the Indian context. It includes understanding idiomatic expressions and context-dependent language use.
For example, taking the case of “ji” rather than purely attaching it to the end of the name, it could be used as an affirmatory phrase meaning “Yes.” Or the phrase "adjust maadi" in Kannada, which loosely translates to 'please adjust' and is often used to ask for understanding or cooperation in various contexts. Implementing this in LLMs requires exposure to a corpus of data that showcase these subtleties.
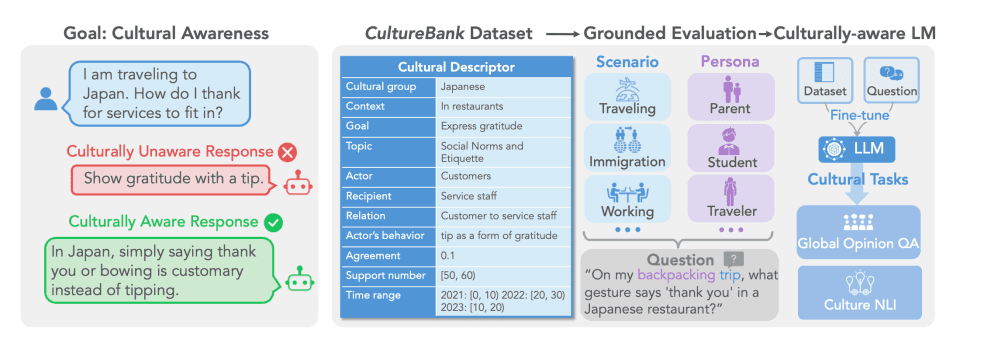
An example of what an adaptive response would look like.
Intuitive (subconsciously learned): This deepest level includes the innate understanding of cultural values, attitudes, and unwritten social norms that Indians typically develop throughout their lives. An example is the concept of "Jugaad," a term used across many parts of India to describe a flexible approach to problem-solving that involves improvisation and ingenuity with limited resources.
But it could also mean taking an easy way out or flirting with lines of ethics in another part. Training LLMs to understand and incorporate such concepts requires advanced machine learning techniques that can interpret and generate responses that reflect these deeply ingrained cultural nuances.
So How Do We Make LLMs Culturally Intelligent?
While this is an area that has garnered significant interest, there is much progress yet to be made. Some methods that stand out are:
Cultural Prompting: A simple but effective solution that's like giving the AI a cultural identity card. Instead of letting it be culturally neutral, specifically telling it to think from an Indian perspective (e.g., "respond as someone raised in a Marwadi joint family in Mumbai") has shown to reduce cultural disconnect by 30-50% across different AI models. While not perfect, it's a practical first step toward making these systems more culturally aware.
Few-Shot Learning: A more practical approach involves enhancing existing AI models using carefully selected cultural examples. Its more cost-effective than building a new model and can be implemented more quickly while maintaining quality.
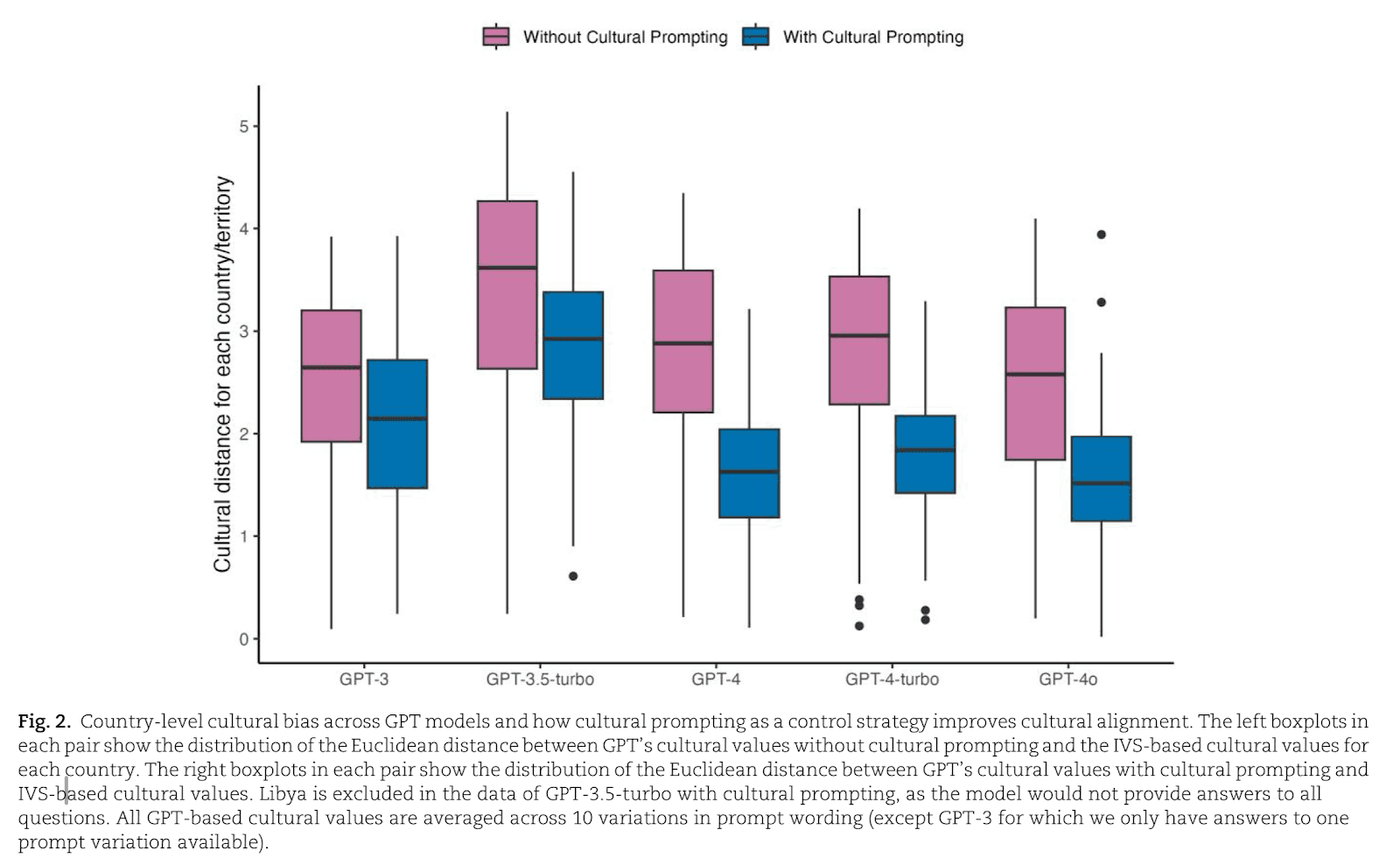
Building Culture Specific Models: A more comprehensive approach involves building culture-specific models, as demonstrated by initiatives like Microsoft's CultureLLM and Taiwan-LLM. This method involves extensive fine-tuning using locally relevant data, including everything from literature and TV shows to social media conversations and regional language content.
Such models can be trained to understand the nuances of different regions –– from Kerala to Punjab, from metropolitan cities to rural villages. While this approach requires significant data collection and computational resources, it offers the most thorough solution for creating AI systems that truly understand our diverse social fabric.
Adversarial Training for Bias Mitigation: A significant challenge towards culturally intelligent LLMs is to reduce the bias arising from the overrepresentation of dominant cultural narratives. Adversarial learning involves training a model with challenging examples to make it more robust and reduce biases.
For instance, if the LLM consistently misrepresents cultural practices, adversarial training can be used to expose the model to these cultural nuances and correct its responses. This approach can be utilised to identify and mitigate biases, thus increasing the fairness of the model in culturally diverse settings.
Call to Action: A Community Effort
At Sukoon, we take a DPI approach to developing solutions across various mental health contexts and building in cultural nuances into our tools and thinking is one of our core focus efforts. We want to create systems that speak authentically to millions of Indians, honouring their language, values, and identity. Click here to learn more about the project.
To make this a reality, we need a coordinated effort from various organisations, NPOs, and NGOs to contribute data that reflects our cultural diversity. This data donation drive would be crucial in building culturally aware LLMs, making mental health support more accessible and relevant for Indian users. If this mission resonates with you and you’d like to discuss further or contribute, get in touch.
About the authors
Tanisha Sheth leads Project Sukoon at People+ai, focused on capacity building within mental health. She also works with several pharma and healthtech companies on product and growth. Tanisha gets excited about the applications of AI to augment care delivery within the industry.
Ambica Ghai is a researcher in machine learning applications. She is passionate about using technology to solve real-world problems and enhance user experiences. Ambica values continuous learning and collaboration to drive meaningful impact.
Ved Upadhyay is an AI solutions architect with 8 years of industry experience designing enterprise systems in domains such as healthcare, pharma, retail, ecommerce, etc. Apart from solving industry problems, Ved actively contributes to academic research through publications in healthcare and Machine Learning, bridging the gap between theory and practical applications. Ved is passionate about leveraging AI to solve real-world problems and create meaningful societal impact.
Special thanks to Vishwa Puranik and Arjun Balaji who helped us with this piece.
References
[1] Y. Tao et al., "Cultural bias and cultural alignment of large language models," PNAS Nexus, vol. 3, no. 9, 2024.
[2] "CultureLLM: Incorporating Cultural Differences into Large Language Models," arXiv, 2024. [Online]. Available: https://arxiv.org/html/2402.10946v1
[3] "Taiwan LLM: Bridging the Linguistic Divide with a Culturally Aligned Language Model," arXiv, 2024. [Online]. Available: https://arxiv.org/abs/2311.17487
[4] Kim, Byungju, et al. "Learning not to learn: Training deep neural networks with biased data." Proceedings of the IEEE/CVF conference on computer vision and pattern recognition. 2019.